ECE Seminar Series – Jun 5 (Mon) @ 2:00pm: "Exploiting Geometric Structure in Machine Learning and Optimization," Melanie Weber, Ass't Prof. of Applied Math & Science, Harvard
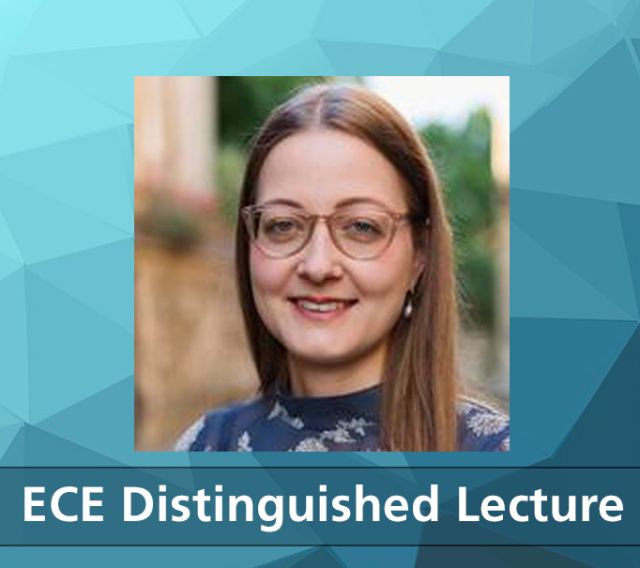
Come at 1:30p for Cookies, Coffee and Conversation!
DISTINGUISHED LECTURE at the ECE SEMINAR SERIES
Abstract
Motivated by the observation that many applications involve non-Euclidean data, such as graphs, strings, or matrices, we discuss how geometric structure can be exploited in Machine Learning and Optimization. First, we discuss the problem of characterizing data geometry from the perspective of Discrete Geometry, specifically focusing on the analysis of relational data. Secondly, we discuss examples of utilizing geometry in downstream tasks. We will focus specifically on problems that involve optimizing functions on manifolds and discuss classes of optimization problems where exploiting Riemannian geometry can deliver algorithms that are computationally superior to standard Euclidean approaches.
Bio
Melanie is an Assistant Professor of Applied Mathematics and of Computer Science at Harvard University. Her research focuses on utilizing geometric structure in data for the design of efficient Machine Learning and Optimization methods. In 2021-2022, she was a Hooke Research Fellow at the Mathematical Institute in Oxford. Previously, she received her PhD from Princeton University (2021), held visiting positions at MIT and the Simons Institute in Berkeley, and interned in the research labs of Facebook, Google, and Microsoft. In addition to her academic work, she is the Chief Scientist of the Legal Artificial Intelligence start-up Claudius Legal Intelligence, where she leads a team of researchers in developing Trustworthy Machine Learning tools for legal analytics.
Hosted by: The ECE Seminar Series
Submitted by: Nina Miolane <ninamiolane@ucsb.edu>