Mar 20 (Mon) @ 10:00am: "Can Kernels be a Viable Alternative to Deep Learning?," Parthe Pandit, Postdoctoral Fellow, UCSD
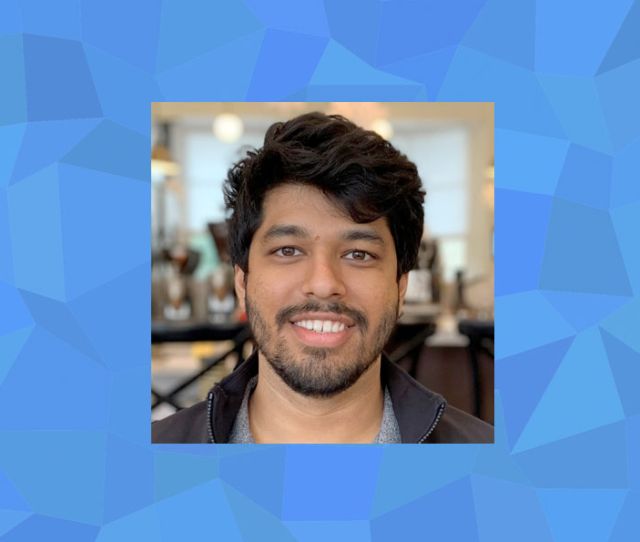
Abstract
Deep Learning has had a tremendous impact on a broad range of applications in science and engineering. Training deep networks however, remains an art with several heuristics that do not translate across application domains. To make Machine Learning reliable and widely accessible, we need to develop principled and stable methodologies.
Kernel methods, a classical model, have received renewed attention following the discovery of the Neural Tangent Kernel and its equivalence to wide neural networks. I will present 2 works which show the promise of kernel methods for modern large scale data analysis applications:
1. Recursive Feature Machines: a new class of adaptive kernel models that learn task-specific features (https://arxiv.org/abs/2212.13881), and
2. EigenPro3: a new iterative algorithm that enables scalable training of large kernel models over large datasets (https://arxiv.org/abs/2302.02605).
Bio
Parthe Pandit is an HDSI-Simons postdoctoral fellow with the Halıcıoğlu Data Science Institute at UC San Diego, and the Simons Institute for the Theory of Computing at UC Berkeley. He obtained his Ph.D. in Electrical and Computer Engineering, and M.S. in Statistics both from UCLA, and a B.Tech. + M.Tech. dual degree in Electrical Engineering with a minor in Computer Science from the Indian Institute of Technology (IIT), Bombay.
His research spans Machine Learning and Signal Processing with a focus on the design and statistical analysis of iterative optimization procedures for high dimensional estimation and inference problems. He has been a recipient of the Jack K. Wolf student paper award at ISIT 2019, and a Distinguished PhD dissertation finalist at UCLA ECE in 2022. He was a research intern with Amazon Search and Amazon AWS working on Natural Language Generation; and with Citadel LLC, working on optimal trade execution and market impact. Apart from Machine Learning, he has also published articles in Graph Theory, Coding Theory, Network Economics, and planning EV Charging infrastructure.
Hosted by: ECE Department
Submitted by: Amy Donnelly <amymdonnelly@ucsb.edu>