Xie & Deng “Combined Intelligence”
Researchers developed a riderless bicycle as a proof-of-concept for the Tianjic chip, which combines the two main types of machine-learning approaches
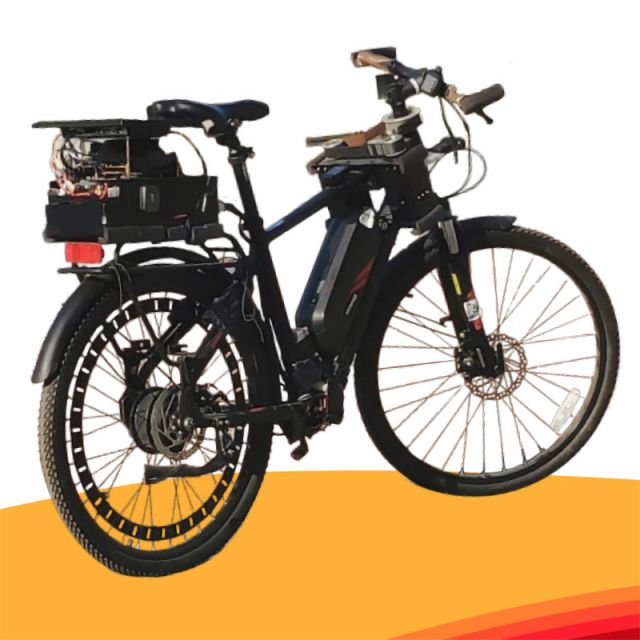
In its rapid rise to prominence, artificial intelligence (AI) has developed, so far, along two main paths: the machine-learning path and the neuroscience path. The machine-learning (ML) path is aimed at bringing a high degree of accuracy to practical tasks — with the help of big data, high-performance processors, effective models, and easy-to-use programming tools. It has achieved human-level (or better) performance in a broad spectrum of AI tasks, from image and speech recognition, to language processing and autonomous driving, etc.
The neuroscience approach is meant to harness what we know about the brain’s neural dynamics, circuits, coding, and learning to develop efficient “brain-like” computing capable of solving complex problems that are not exclusively data-driven and may involve noisy data, incomplete information and highly dynamic systems.
The two types of AI use different approaches to solving these different types of problems, and, not surprisingly, both are based on different and wholly incompatible software models, or platforms. Neuroscience AI takes place in what are referred to as spiking neural networks (SNNs), while machine learning occurs in the slightly misleadingly named (because of the word “neural”) artificial neural networks (ANNs).
“The Neuroscience [NS] approach mimics the behavior of our brain circuits,” says Lei Deng, a postdoctoral researcher in the laboratory of UCSB computer science professor Yuan Xie and a co-author of a recent paper in Nature titled “Towards artificial general intelligence with hybrid Tianjic chip architecture.”
He adds, “We know that our brain can perform many tasks better than a computer can. The problem in terms of NS-oriented AI is that so many details of how our brain works are still unclear. As a result of those gaps in knowledge, we can say that the CS approach is very successful now, but the NS model will be the future.”
In the human brain, the inputs for one neuron come from the firing activities of previous neurons, “So, it builds,” says Deng. “A time factor is involved, so the historical states of a neuron will affect its future.
The CS model, on the other hand, does not build upon prior knowledge over time. Rather, it has a database and uses strong computing power to search through the data at high speed to refine matches. But it cannot accumulate knowledge to “learn as it goes.”
“Sometimes, in the human brain, if a neuron accumulates information and the membrane potential crosses a threshold, the neuron will fi re,” Deng says. “But if the stimulus information is not strong, it will not cross the threshold and will be leaked; the membrane potential will decay if no other inputs are received. This process can help to denoise very noisy data. Our brain is a noisy system that receives many partial, ‘noisy,’ signals but is very good at filtering out that noise to extract only what is useful.”
At this stage, and until the brain is better understood and computer scientists can achieve a blended artificial general intelligence (AGI), Deng says, “We believe that combining these two approaches is a promising path. Our big idea is that if we want to go a step further at this stage, we should build a cross-paradigm computing model.”
It is a challenging proposition, as, usually, ANNs and SNNs have different modeling paradigms in terms of information representation, computation philosophy, and memory organization. Deng and his colleagues have addressed those issues to some extent for the first time on their Tianjic chip, which, as the authors write, “integrates the two approaches — computer-science-oriented and neuroscience-oriented neural networks — to provide a hybrid, synergistic platform.”
COE/CLS Convergence (F19) – “Combined Intelligence” (full article pg. 30)