Interdisciplinary Team
Professor William Smith from UCSB's Department of Molecular, Cellular and Developmental Biology (EEMB) teams up with ECE professor B. S. Manjunath and his student Angela Zhang in an interdisciplinary undertaking
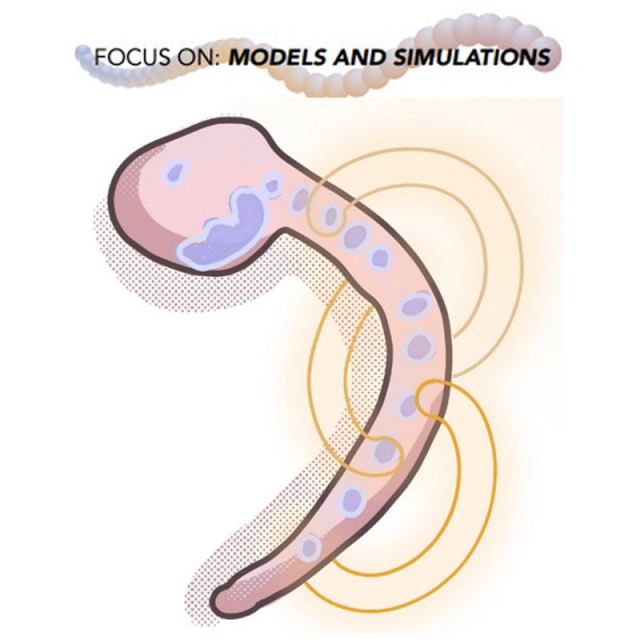
W. Smith, Manjunath & A. Zhang | "The Ciona, Model Organism"
Excerpt From the COE/CLS Convergence magazine (F/W21) article "Focus On: Models and Simulations"
William Smith understands as well as anyone that mapping the neural circuitry of the human brain and tracking the coordinated interactions of our roughly 85 billion neurons is a daunting task.
"Ultimately, we’d like to understand how vertebrates, animals like us, work," says Smith, whose research is focused on the nervous system of animals in the chordate phylum of animals, which includes vertebrates. "But we’re enormously complicated, and at this point we’re very far from a full understanding of our complex nervous system at the individual neuron level; there are just too many neurons."
A better place to start, he thought, would be to model the neural circuitry of a simpler chordate organism, to serve as a surrogate for — a kind of model of — the human neural system. Enter the ocean-dwelling Ciona, a primitive chordate that is closely related to vertebrates but has only 177 neurons. As one of few universities to have marine science facilities on its main campus, UCSB is ideally situated for this research.
The work, funded by NIH’s Institute of Neurological Disorders & Stroke, began with Smith’s collaborators at Dalhousie University in Halifax, Nova Scotia, gathering electron microscopy (EM) images of every neuron in a Ciona larva and then creating a wiring diagram, or connectome. Even with so few neurons, the project took many years of work by the Dalhousie team. Their results can be seen as an interaction matrix showing the 6,618 synaptic connections among the 177 neurons. "To do that for the human brain would require a matrix 2.17 x 1017 — or a billion trillion — times bigger than that," Smith says.
Beyond mapping the Ciona’s neural circuitry, the researchers wanted to determine which of six key neurotransmitters are present in which neurons. "It’s important to know, because that tells us how it will act on its targets. Neurons can be excitatory — they can tell other neurons to have an action potential downstream — or they can be inhibitory," Smith says. "It’s not a trivial undertaking."
To begin that work, Smith teamed up with UCSB Electrical and Computer Engineering professor B. S. Manjunath, Co-PI on the NIH project and an expert in computer vision and machine learning, and his student Angela Zhang, who received her PhD in September 2021, in an interdisciplinary undertaking of the kind that is much valued at UCSB. Zhang began by developing a method for overlaying the EM data onto the light microscopy (LM) data from Smith’s lab, bringing the two kinds of information into alignment, or register, so that specific organs in the Ciona, seen with LM, could be correlated with specific neurons identified in the EM data.
The light microscopy was conducted using in situ hybridization, which involves incubating fixed Ciona larvae with fluorescently tagged antisense RNA probes. Each RNA probe differs depending on the type of neurotransmitter marker it is meant to find, and when the probe locates the target, it fluoresces in a color correlated to that neurotransmitter.
The light microscopy allowed Smith’s group to do a three-dimensional reconstruction of the actual nervous system, but not at synaptic resolution, which requires electron microscopy. "We can see the outlines of cells here, but not all the little fine synapses," he says, pointing to a photograph of an LM-generated image.
"Maybe we know the nucleus location of a cell in both sets of data. We have the shape information of this cluster of cells from the EM and of another cluster of cells from the light microscopy," Zhang says. "I have to use computation to rotate and stretch and kind of move the cells in one set of data so that it overlays well with the other set. If we get the alignment right, we can see how a cell in the EM data corresponds to one in the LM data."
The registered data can then be correlated with the location of neurons to deduce which neurotransmitters might be present at specific synapses. "We got pretty good matches for certain parts of the brain, such as the photoreceptors," Zhang says. "We could match the location of the neurons in the two sets of data."
Smith’s team could then use logic and their own eyes to go further, for example, identifying specific neuron types. "We know from the EM data that there is a cluster of cells located by itself, between the head and the tail, and that it is emitting only one type of neurotransmitter, GABA [the inhibitory neurotransmitter gamma-aminobutyric acid], for example, because the probe is lighting up with the color associated only with GABA."
Things were less resolved in other locations. "They’d see something that looked kind of like a hand and fluoresced in many different colors," Zhang explains. "They knew the location of the cell and its nucleus from the EM data, but the intermingled colors of fluorescence prevented them from knowing what was going on. But, by combining many samples, we were able to make predictions with high confidence for most neurons."
Smith says that by allowing his team to model what kinds of signals are sent between neurons, Zhang’s initial work "led to some new and interesting models about how the nervous system in this animal works," for example, that many of the behaviors in Ciona are evoked by disinhibition, or one inhibitory neurotransmitter inhibiting the (inhibitory) influence of another inhibitory transmitter, thereby allowing an action to occur.
After converging the LM and EM data, he adds, "Angela would come up with a hypothesis, saying maybe, 'I think these cells are inhibitory and connect with other cells that also appear to be inhibitory,' and then we’d do various behavioral and pharmacological experiments that would allow us to say, 'Yes, that really is how it works.' It’s modeling, where we’re working together."
Next, Zhang built a deep-learning model to try to complete the work of correlating specific cells with their neurotransmitter types. The in situ hybridization had made it possible to identify with confidence some neurons and the various neurotransmitters they make. She used those confirmed data points to train the model, providing it with examples of known excitatory and inhibitory synapses. She thought, What if we used the EM data to look at the individual synapses and see if there are physical differences in their structures that can indicate whether it is an excitatory or inhibitory synapse?
One challenge, Zhang says, was that, unlike many engineering problems, where the final answer is known and the task is to train an ML model to get the right answer, "With science, you don’t actually know what the truth is; you’re trying to figure it out."
The result, she says, is that "The model is able to learn, but we don’t know what it is learning. Is it learning that a particular set of photoreceptors has a certain quirk, so it groups them together? Or is it learning something universal to all excitatory Ciona synapses? And can we trust the results of the model? We can’t know now without more experiments, and I’m definitely not an advocate of trusting models blindly."
As Smith likes to say, "All models are wrong. Some are useful." Time will tell.