Miolane – NSF Grant
ECE Ass't. Prof. Nina Miolane receives an NSF grant of $334,780 (3 years) for her research project that examines the need to rigorously understand and expand the data types to which deep-learning methods can be applied
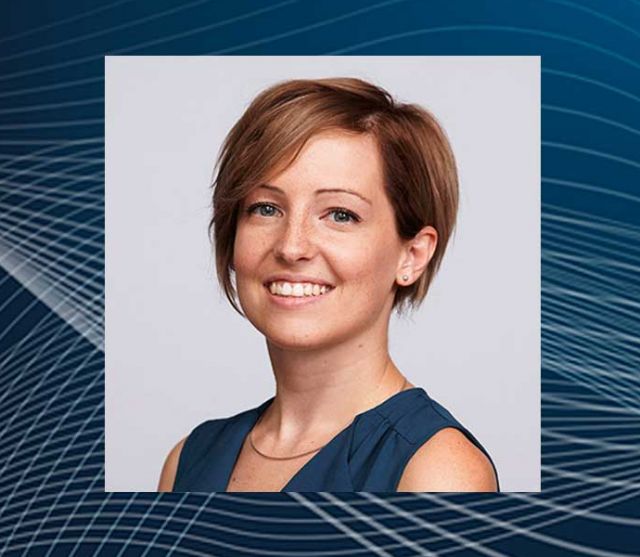
Nina Miolane | "A Unifying Deep Learning Framework Using Cell Complex Neural Networks"
Excerpt from the COE/CLS Convergence magazine (F/W21) article "New Grants"
Deep learning has fostered the development of many new transformative technologies that originated with rapid advancements in the fields of computer vision and natural language processing, that is, the processing of images and texts. Yet, a wide range of data is not best represented by a grid of pixels or a sequence of words. For example, (biomolecular) shapes and (social) networks are data types exhibiting local and global geometric properties that might not be efficiently leveraged by existing deep-learning architectures. Hence, there is a need to rigorously understand and expand the data types to which deep-learning methods can be applied.
This research project considers the more abstract "cell complex" data type, and is aimed at quantifying the potential of "cell complex networks" in deep learning. Applications range from computational biology and medicine, social science, and art, to a better understanding of deep learning itself. Nina Miolane will collaborate with Mustafa Hajij, an assistant professor of mathematics and computer science at Santa Clara University.
COE/CLS Convergence magazine (F/W21) - "New Grants" (pg. 36)