Pedarsani – Joint ComSoc / ITSoc Paper Award
Prof. Ramtin Pedarsani's award recognizes outstanding papers that lie at the intersection of communications and information theory
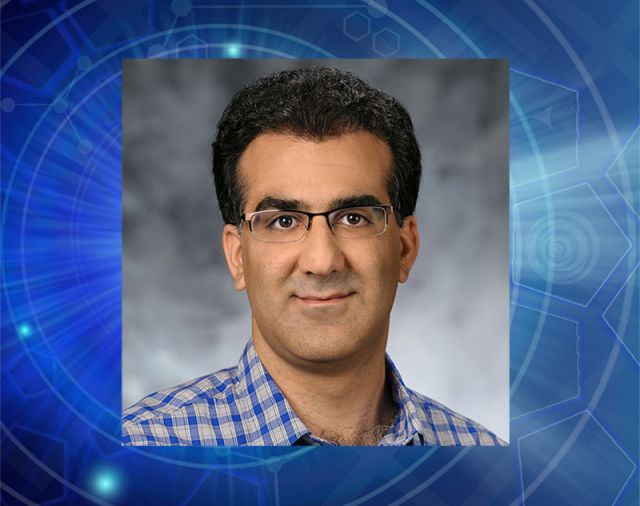
The Joint Communications Society/Information Theory Society Paper Award Committee, made up of members from both societies, gave the 2020 award to Kangwook Lee, Maximilian Lam, Ramtin Pedarsani, Dimitris Papailiopoulos and Kannan Ramchandran for their paper "Speeding up Distributed Machine Learning Using Codes", IEEE Transactions on Information Theory, vol. 64, no. 3, pp. 1514-1529, March 2018.
In recent years, the computational paradigm for large-scale machine learning and data analytics has shifted towards massively large distributed systems, comprising individually small computational nodes. In large-scale systems there are several types of "systems noise" that can affect the performance of distributed machine learning algorithms including systems heterogeneity and communication bottlenecks. Their paper was the first to introduce the fundamental role of coding theory in distributed machine learning, and how it can provably achieve significant speed-up gains. As a result of this highly-cited paper, a new research area called "Coded Computing" has emerged in the information theory and machine learning communities.
The Pedarsani Group's research overview is on distributed machine learning (ML) systems that can enable a variety of new applications in health-care, autonomous vehicles, smart cities, robotics, etc. However, distributed ML faces several systems and algorithmic challenges including communication bandwidth, systems heterogeneity, data heterogeneity, adversarial perturbations, and scalability. Their research leverages tools from optimization theory and information theory to tackle these challenges, and develop provably efficient algorithms with strong statistical performance guarantees for the machine learning model. In particular, they aim at developing fast, robust, and private federated learning and collaborative learning algorithms that achieve optimal performance in terms of convergence rate for both convex and non-convex settings. In another project, they focused on human-cyber-physical-systems (h-CPS) such as next-generation transportation systems that involve both human and robot agents. Their research leverages tools from reinforcement learning and game theory for data-driven efficient control and management of h-CPS.
Ramtin Pedarsani is an assistant professor in the ECE department at UCSB. He obtained his Ph.D. in Electrical Engineering and Computer Sciences from UC Berkeley in 2015. He received his M.Sc. degree at EPFL in 2011 and his B.Sc. degree at the University of Tehran in 2009. His research interests include machine learning, optimization, information and coding theory, and stochastic networks. He is the recipient of the Communications Society and Information Theory Society Joint Paper Award in 2020, the best paper award in the IEEE International Conference on Communications (ICC) in 2014, and the NSF CRII award in 2017.